About Halicin
On February 20, 2020, the journal Cell published “A Deep Learning Approach to Antibiotic Discovery,” a paper on the discovery of the super-powerful antibiotic Halicin by the research team of MIT bioengineer James Collins, using deep learning models. Halicin, as it is called, is named after Hal 9000, the AI system from the movie 2001: A Space Odyssey.
Halicin was initially studied for the treatment of diabetes, but the program was not further developed due to poor test results. However, thanks to advances in AI deep learning, Halicin is now available for better use.
Halicin kills bacteria by disrupting the electrochemical gradient across the cell membrane of the bacteria. This gradient is necessary for the production of ATP, the molecule that cells use to store energy. Therefore, if the electrochemical gradient is disrupted, the cell will die. In addition, this killing mechanism makes it difficult for bacteria to develop resistance to the drug.
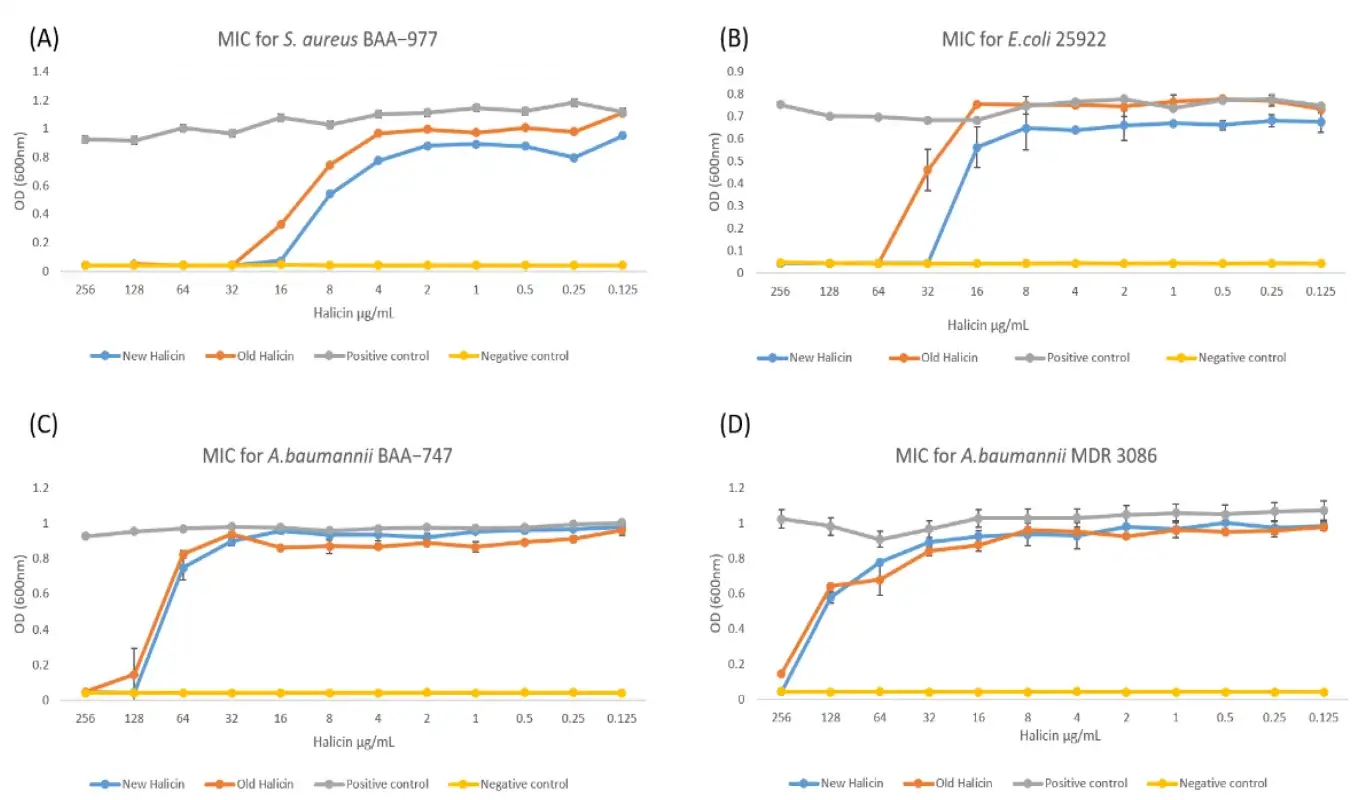
The results showed that Halicin was bactericidal against Clostridium difficile, Acinetobacter baumannii and Mycobacterium tuberculosis, in addition to Pseudomonas aeruginosa, a Gram-negative bacterium susceptible to lung infection.
In one study, E. coli did not develop any resistance to Halicin during a 30-day treatment period. In contrast, these bacteria became resistant to Ciprofloxacin (another antibiotic) within 1 to 3 days, and after 30 days, these bacteria were about 200 times more resistant to Ciprofloxacin than at the beginning of the trial.
To test the efficacy of Halicin in animals in vivo, researchers used it to treat mice infected with Acinetobacter baumannii, a multi-drug resistant strain of bacteria. After 24 hours of applying Halicin ointment, the mice were completely cleared of the infection.
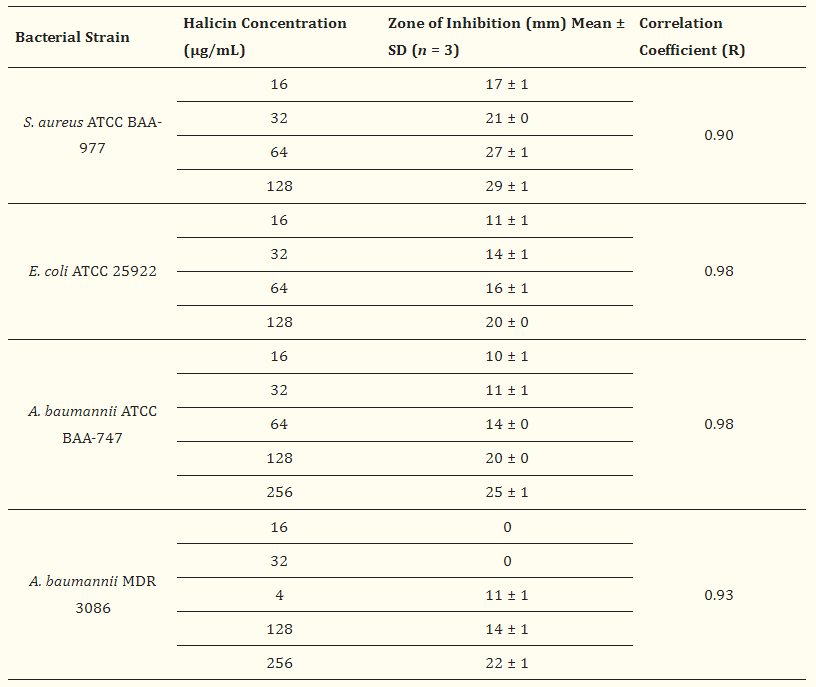
AI algorithm and filtering
Currently, the discovery of antibiotics is mostly initiated by screening large synthetic chemical libraries. However, the time and administrative costs of searching through large libraries are often prohibitive. By contrast, the discovery of Halicin is much more efficient.
Halicin was developed using Deep learning in Machine learning, a model that learns the structure and specific chemical groups of different drugs and can predict the properties of the molecules. The simple steps are as follows:
- We collected 2335 molecular structures from the database and tested the compounds for growth inhibition of E. coli. The effective compounds were labeled as 1 and the ineffective compounds were labeled as 0. The data were then put into a deep training model.
- The Drug Repurposing Hub identified 6,111 molecules that were dropped directly into the model to predict the effect, with a score closer to 1 indicating a greater effect. The top 99 compounds were selected for cell testing and 51 of them were found to be effective. The 63 molecules with the lowest scores were also subjected to additional cell testing, and only two of them showed partial effects.
- Halicin was selected considering the structure of the compound and the ease of synthesis, and was then tested on rats infected with a variety of drug-resistant bacteria.
Using this system, the screening process has been dramatically shortened. “This groundbreaking work signifies a paradigm shift in antibiotic discovery and indeed in drug discovery more generally,” said Roy Kishony, professor of biological and computer sciences at the Technion-Israel Institute of Technology.
Subsequent optimization of the molecules
After determining the structure of Halicin, the researchers screened the ZINC15 database🔗 (out of more than 100 million molecules) for 23 candidates that were structurally different from existing antibiotics and were predicted to be non-toxic to human cells. Amazingly, the screening took only three days.
The 23 candidate molecules were tested against five species of bacteria, and the researchers found that eight of them showed antibacterial activity, and two were particularly strong. The researchers plan to further test these molecules and will also screen more of the ZINC15 database.
This approach will allow deep learning to be applied to all stages of antibiotic development, including drug modification, improved efficacy and toxicity.
Current Difficulties in Antibiotic Development
Drug resistance
By 2050, the number of deaths from drug-resistant infections will reach 10 million per year. If research and development does not keep up with the rate of bacterial change, more and more patients with drug-resistant infections will be left without a cure.By 2050, the number of deaths from drug-resistant infections will reach 10 million per year. If research and development does not keep up with the rate of bacterial change, more and more patients with drug-resistant infections will be left without a cure.
“We’re facing a growing crisis around antibiotic resistance, and this situation is being generated by both an increasing number of pathogens becoming resistant to existing antibiotics, and an anemic pipeline in the biotech and pharmaceutical industries for new antibiotics,” Collins says.
AI Learning
The above deep learning model using E. coli as the prediction score seems to be not comprehensive enough, maybe we can try to test with other bacteria or add more discriminators in the future. Furthermore, the absence of good pharmacokinetic parameters in the database may lead to poor prediction of the compounds’ pharmacokinetics. Even if the predicted structures are available, they may not be easy to synthesize, and these are the parts to be improved in the future.
Conclusion
The invention of antibiotics has brought benefits to human medicine, but over-abuse has also led to serious resistance. How to find a balance between antibiotics and disease, and to develop more effective antibiotics, depends on how human beings use the wisdom of AI.
Articles about AI or antibiotics:
References:
- Booq RY, Tawfik EA, Alfassam HA, Alfahad AJ, Alyamani EJ. Assessment of the Antibacterial Efficacy of Halicin against Pathogenic Bacteria. Antibiotics (Basel). 2021;10(12):1480. Published 2021 Dec 2. doi:10.3390/antibiotics10121480 🔗
- Jonathan M. Stokes, Kevin Yang, Kyle Swanson, Wengong Jin, Andres Cubillos-Ruiz, Nina M. Donghia, Craig R. MacNair, Shawn French, Lindsey A. Carfrae, Zohar Bloom-Ackermann, Victoria M. Tran, Anush Chiappino-Pepe, Ahmed H. Badran, Ian W. Andrews, Emma J. Chory, George M. Church, Eric D. Brown, Tommi S. Jaakkola, Regina Barzilay, James J. Collins,A Deep Learning Approach to Antibiotic Discovery,Cell,Volume 180, Issue 4,2020,Pages 688-702.e13,ISSN 0092-8674,https://doi.org/10.1016/j.cell.2020.01.021. 🔗
- Yang, K., K. Swanson, W. Jin, C. Coley, P. Eiden, H. Gao, A. Guzman-Perez, T. Hopper, B. Kelley, M. Mathea, A. Palmer, V. Settels, T. Jaakkola, K. Jensen and R. Barzilay (2019). “Analyzing Learned Molecular Representations for Property Prediction.” J Chem Inf Model 59(8): 3370-3388. 🔗
- MIT News: Artificial intelligence yields new antibiotic: A deep-learning model identifies a powerful new drug that can kill many species of antibiotic-resistant bacteria. 🔗
- Image taken from pixabay